AI Passion Isn’t a Soft Skill: Ditch AI Enthusiasm From Job Ads
- Anna Tarasiuk
- Feb 14
- 6 min read
Updated: Feb 18
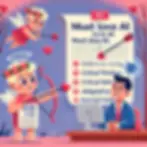
While most companies don't explicitly seek "AI enthusiasts," job postings demanding candidates "passionate about all things AI" are not uncommon. Understandably, employers want to hire professionals who can skillfully leverage AI tools. But insisting on a passion for AI? Come on, let's be reasonable.
My passion for coffee borders on an addiction; I cannot imagine a morning without a strong brew that’s at least 70% arabica and 30% robusta. I anxiously anticipate the aroma and the feel of a hot (one-and-only, coffee-exclusive) cup in my palms. But for some reason, I just don't get the same vibe for a coffee maker. Or running water. Or electricity, for that matter.
My emotional disregard for technological wonders aside, let's rewind a few years to the days before the AI boom. Can you imagine a job description for an SEO manager who must necessarily be passionate about Google Keywords Planner? Or Ahrefs? Probably not.
Now, AI enthusiasts may argue that ‘AI-passionate’ as a soft skill shows that a person is open-minded, ready to embrace change, and highly adaptable.
It doesn't.
Especially considering that the current state of AI adoption is already past the ‘enthusiasm’ stage. And there is rational proof to justify this claim.
To illustrate why “AI passion” is overrated, let’s look at two classic models: Rogers’ Technology Adoption Lifecycle (to understand the current state of AI adoption in the workplace) and Gartner's Hype Cycle (to see how public perception and expectations evolve over time).
Technology Adoption Lifecycle by Everett Rogers

The model explains how every new technology is adopted by society:
Innovators (2.5%), the first to adopt new tech
Early Adopters (13.5%), visionaries who see potential early
Early Majority (34%), pragmatists who adopt once benefits are proven
Late Majority (34%), skeptics who wait until the tech is established
Laggards (16%), resistant to change or limited by external factors
Innovators are always the first to adopt any new tech, followed shortly by early adopters. Then, the stakes go up because technology must cross the chasm between early adopters and the early majority. Many products cannot manage this, but AI can.
Quoting ChatGPT, AI is crossing the chasm right now:
As of 2025, AI is moving from niche early adopters to widespread business and consumer use. AI tools are now common in workplaces, customer service, marketing, and creative industries.
Right now, it’s clear that AI will make that crossing. The early majority is catching up already. It’s only a matter of time before the late majority and laggards follow.
That is, the early hype days are almost done. Understandably, most startups are in their innovative and early adoption days themselves, age- and mindset-wise. So, they are targeting employees with similar mindsets; that is, innovators and early adopters. And that's the most reasonable thing to do, but not when AI usage is concerned.
Even if a professional (say, a writer, a designer, or even a developer) was an innovator or an early adopter 2-3 years ago, a single year of daily professional exposure kills the novelty hype. And it’s absolutely fine.
An architect does not need a passion for AutoCad or its newly integrated AI features to love his job, which is designing beautiful, functional homes tailored to each family’s needs.
However, architects certainly appreciate AI-powered modeling and calculation capabilities, which allow them to focus on the truly creative side of the job they've chosen. So do web designers. And writers. And marketers.
Companies that prioritize the candidate’s unrestrained joy about AI capabilities over years of expertise in a given field (to say nothing of time-proven commitment) are unwise. And that’s putting it mildly.
Especially if we consider that the early adoption stage is drawing to a close. If your candidate already has experience working with AI tools, that candidate is definitely not a laggard.
If there is one sign of laggard reactiveness, it's overreliance on specific tools, even versatile ones like AI. Today, tools are evolving quickly, AI especially so. This means there are always new perks and functionalities to master.
A person who's only starting to get excited is already a bit behind. Or simply has very little professional experience. As AI tech is officially entering the early majority user group, enthusiasm is no longer part of it; instead, pragmatism kicks in. GenAI is actively making its way to the ‘nothing personal, strictly business’ phase.
Roger’s model explains technology adoption rates and does a pretty accurate job as far as previous innovations go. In contrast, our next social model focuses on public perception and hype. It would be hard to deny that AI is a hyped topic, so let’s analyze it from the Gartner Hype Cycle perspective.
The Gartner Hype Cycle

The Gartner Hype Cycle describes the journey of emerging technologies as they travel from overhyped excitement to realistic productivity. Now, this model is way more controversial and faces plenty of criticism, but it fits with a minor stretch in the AI context. When asked about its current status based on the Hype Model, ChatGPT's response is
In 2025, AI is likely in the "Slope of Enlightenment" phase of the Hype Cycle, progressing from the Trough of Disillusionment but not yet fully reaching the Plateau of Productivity.
Now, the question is: if there is still so much hype, when did the Trough of Disillusionment happen? For this, we have to go back to the real beginning because AI did not emerge in the 2020s; it has a much longer history of ups and downs.
Innovation Trigger → Peak of Inflated Expectations
1956: Dartmouth Conference workshop, known as the birth of AI
1960s: early AI Research & chatbots like ELIZA
1980s: expert systems boom, including AI use in medical diagnosis and automation.
1990s: machine learning resurgence, the emergence of AI applications in finance, speech recognition, and robotics.
2012: deep learning breakthrough, or modern AI trigger.
Peak of Inflated Expectations → Trough of Disillusionment
These periods are also called AI winters; the technology looked like a lost case to many during the first two.
1973-1980: AI failed to meet expectations of human-level reasoning and natural language processing.
1987-1997: systems proved too expensive and unreliable.
2022 – 2024: practical limitations and regulatory concerns around AI. (Anyone who missed this part was not paying enough attention.)
Trough of Disillusionment → Slope of Enlightenment
That’s where we are right now, and this time, it looks like AI will finally climb that slope of enlightenment and reach the last stage:
Plateau of Productivity
We’re expected to reach this stage by 2030. Possibly, there won’t be any rebounds this time; at least, the tech will unlikely hit the rock-bottom level of previous AI winters.
Overall, AI travels the Garnter stages — not linearly, as the model suggests, but spirally, making round after round.
Companies that still treat AI from the ‘Peak of Inflated Expectations’ perspective (because what else can explain their passion for AI-enthusiastic applicants?) are up for an unpleasant surprise. Or, in this case, the Trough of Disillusionment.
Adopting the hype approach when integrating AI into business operations and hunting for ‘enthusiasts’ shows a limited understanding of the technology and the path it has already traveled. Which is a paradox. At least if we assume that enthusiasm should be backed by basic knowledge and common sense.
AI Enthusiasm Not Required (based on o1 reasoning)

That's enough human reasoning for one post, so let's just ask AI what it ‘thinks.’
Fluff-abridged version? You don’t have to love AI to leverage it.
It’s a tool: proficiency doesn’t require passion; people can use AI in a task-oriented way.
Practical focus: jobs rely on a combination of AI outputs and industry know-how, with industry knowledge a priority.
Routine tasks: much AI work, like data entry, is a routine process that does not allow for creativity or “passion.”
Transferable skills: critical thinking, communication, and project management matter more than AI excitement.
Fast-evolving tech: adaptability beats enthusiasm for any single tool.
Diverse roles: not everyone on the team needs to “live and breathe” AI to use its outputs.
Emphasis on results: quality work and results matter more than personal captivation by AI as a concept.
Avoiding overhype: overenthusiasm can lead to hype that isn’t always rooted in practical understanding.
HURRAY for point number 8, already analyzed from the perspective of the Gartner Hype model!
But jokes aside, it's not funny when job applicants are expected to show (fake?) boundless delight with AI as a concept. Most active professionals now understand that AI is a fascinating, complex technology with huge practical potential in many applied spheres.
It's awesome – both in the modern sense of the word and its original ‘amazingly intimidating’ meaning. But its applied purpose in the professional setting is still that of a tool.
Don't expect carpenters to love their chisels and screwdrivers — they like and appreciate those well enough already. Instead, let them love the beautiful furniture they make and the creative process it entails, no matter which tools they rely on.
Similarly, professional success in the AI era hinges on adaptability, creativity, and deep industry knowledge, not boundless enthusiasm for the tech itself.
Disclaimer: parts of this post, as required by logic and human reasoning, had to be generated with AI, along with several images. Further, the initial text version underwent a series of AI-powered post-edits and spelling checks.
Not so much with passion, but certainly with restrained curiosity.